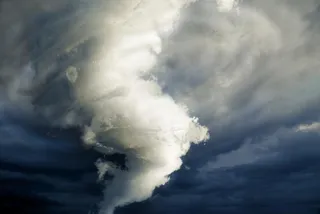
The evolving face of cat models
The world of risk modelling is constantly changing. New dangers are identified, new perils mapped, new areas of vulnerability discerned.
Before Mount St Helens erupted in 1980, volcanic ash fall wasn’t seen as much of an insurance peril—now it is. Flood modelling seems to change after every major flood, especially when global climate change brings a new element to it. Hurricane and windstorm modelling is pored over before, during, and after every hurricane and typhoon season as the insurance industry remembers Katrina, Rita and Wilma—and winces.
In the past 10 years there have been a string of natural catastrophes that have resulted in some fundamental changes to the modelling industry. More risks are now being modelled as the industry learns the lessons of the past.
Drought-afflicted areas that are then hit by flooding see water react in a different way from areas that are already saturated with water. Hurricanes can have a greater impact on regions suffering from coastal erosion. And terrorism is always throwing up unpleasant surprises.
Bermuda:Re+ILS asked industry experts to look at two areas of constant interest for insurers—windstorms and terrorism—and to explain how things have changed in recent years. Here are their findings.
New methods of modelling wind
Windstorms account for the biggest losses in Europe. Alexandros Georgiadis, Patrick Daniell and Aidan Brocklehurst from Impact Forecasting’s windstorm team, examine how new methodologies have changed the way this peril is modelled.
Windstorms cause the highest amount of insured losses in Europe so it is essential for insurers and reinsurers to address the following questions: what is the chance of a recent high impact event occurring again in the future? And what is the impact of the worst-case scenario?
The main purpose of catastrophe modelling is to address such questions. With this aim windstorm models have evolved dramatically over the last decade, largely due to increased collaboration between the insurance industry and academia.
A key component of any probabilistic model is a large, typically synthetic, yet realistic database of catastrophic events covering a long period of time, usually thousands of years. Such long series of events (far larger than any available historic record) are essential for an insurer or reinsurer to obtain statistically robust results that can support important decisions relating to the risk appetite and the required level of protection.
Data sources
In the case of European windstorms, the primary data source for all catastrophe models is historic storm activity. On the European scale, high quality wind data and storm records are available for roughly 50 years, with considerable variation, in quantity and quality, between countries and regions.
Early windstorm catastrophe models (from the mid-1990s) developed probabilistic event sets based purely on these historical records. Key meteorological parameters of past events such as the recorded wind speeds and the storm-tracks were statistically sampled, often using Monte Carlo methods, to build new synthetic storms.
Typically the final product was a probabilistic set of storms of varying intensities, spanning tens of thousands of years, spawned from the past 30 to 50 years of storm activity. These early models had the limitation that they relied on purely statistical approaches to generate extreme impact events.
A significant development regarding the generation of synthetic storms was the introduction of events based on the output of numerical weather prediction (NWP) models. This methodology uses ensemble forecasts to create multiple possible realisations of historic storms, which can be more or less severe than the historical outcome. It has the advantage of generating physically realistic synthetic storms in terms of track and intensity, based on the NWP’s ability to capture the dynamics and the evolution of the atmosphere.
Each NWP model ensemble may have up to 50 possible realisations of the same storm. As a consequence, using a few years’ (even decades’) worth of the forecast outputs allows model developers to create stochastic event sets with thousands of different (yet related) synthetic events. Each one of these synthetic storms is a physically plausible perturbation of a historical storm.
Nevertheless, this approach has two important limitations:
• Rare events: NWP-simulated realisations are still constrained by historic occurrences seeding them and thus cannot easily provide a view on the threat of rarer weather types; and .
• Windstorm clustering, the phenomenon whereby severe windstorms often occur in close succession within the same season (eg, during the 1999 and 1990 winter seasons). In the past these synthetic storms were grouped into probabilistic years using different assumptions to give a statistical rather than a physical view of clustering; recent academic work has suggested however that statistical methods may overestimate the potential for large events to cluster.
Recent developments
The most recent major development in windstorm modelling involves the use of global circulation model (GCM) outputs. These are mathematical models, typically using the same suite of physical equations as the NWP models to simulate the entire earth system and its climate evolution.
They are able to capture the dynamic behaviour, evolution and interactions (such as mass and energy flows) between the land, the ocean, the atmosphere and the cryosphere (ice-sheet, ice-shelves, glaciers). Their output includes continuous simulations of the state and evolution of the atmosphere over many decades or centuries.
Due to the long time periods covered and the extensive domain (the entire earth system) involved, these simulations usually run at low resolution (grid size of 200km). Thus, storms extracted from the atmospheric output of the GCMs usually need to be downscaled to higher resolution (5-10 km grid size) to provide synthetic events for probabilistic windstorm modelling. Current downscaling techniques can also be aided by the use of NWP modelling. The resulting gust intensities can be calibrated against a historic record on a grid point basis.
This approach addresses the two key shortcomings of using only NWP models as described above. First, rare weather types can be modelled using GCMs. The simulation of the earth system from a GCM allows for exotic (yet physically plausible) storm types or storm sequences to be generated, and implemented in the stochastic event set.
Second, the GCM output provides entire storm seasons as a continuous simulation, in which varying numbers of storms occur, hence clustering is inherently included in the model and synthetic years do not have to be reconstructed statistically.
Changing views
Looking beyond the technical developments, progressive changes in the views and attitudes of the modelling community are also evident over the last few years. GCMs are highly complex tools, requiring extensive computing and scientific resources. They represent the cutting edge of climate research and are typically developed, maintained and managed by academic/research entities such as the Max-Planck Institute in Europe and the National Oceanic and Atmospheric Administration (NOAA) in the US.
The use of these tools in modelling within the insurance industry naturally requires and promotes increasing collaboration between the industry and academia and encourages greater transparency within model development.
Transparency benefits model users, insurers and reinsurers as it provides them with a much greater understanding of the modelling, which in turn allows them to take far greater ownership of their own risk.
A decade of development
Terrorism modelling has come a long way since RMS released the industry’s first model in 2002, as Chris Folkman, director, terrorism risk and model product management, RMS, explores.
Today’s models provide data-driven insights into the risk, enabling insurers to more accurately price and underwrite terrorism risk, purchase reinsurance that matches a portfolio’s risk profile, and manage capital to avoid the inherent severity of terrorism losses. These advancements have contributed to today’s robust global terrorism insurance market, with adequate capacity and take-up rates of more than 60 percent in the US.
Models have come a long way
The September 11, 2001 terror attacks upended the insurance industry. Approximately 10 percent of the US property & casualty surplus evaporated overnight, sending a shock wave through the insurance and reinsurance markets and resulting in a sudden, severe capacity shortage.
At the time, terrorism was not explicitly accounted for in pricing and underwriting. Carriers had little detailed information on the concentrations of their insured risk and there was almost no standard contract language addressing acts of terror in property coverage.
Terrorism began to be excluded by most commercial insurance policies almost immediately afterwards. The lack of available coverage stalled large construction projects and led to the downgrade of $4.5 billion of commercial securities.
Two things helped resuscitate the private market for terrorism insurance. First, President George W. Bush signed the Terrorism Risk and Insurance Act (TRIA) into law, enabling broader availability of coverage. Second, catastrophe models for terrorism risk were released in 2002, providing a better understanding of the likely damages resulting from large-scale acts of terrorism.
If today’s terrorism models can be likened to a surgeon’s scalpel, the ones released in 2002 and 2003 were cudgels. In an effort to quickly provide insurers with a much-needed tool for evaluating terrorism risk, the models were rapidly constructed, delivering a rough approximation of the risk of large-scale attacks. Although basic, they were a valuable frame of reference for insurers.
During the next decade, models improved as more data became available, blast dynamics were more closely studied, and terrorism became more widely understood. The most recent version of the RMS terrorism model incorporates data on more than 125,000 historical attacks, hundreds of known plots, and dozens of global threat groups. Unlike a decade ago, terrorism modelling is a data-driven undertaking.
Insurers use terrorism models in three ways
First, for accumulation management purposes. Insurers and reinsurers closely monitor their concentration of exposure (property value, or people, or both) in high-risk urban areas, in the proximity of high profile buildings. Geospatial tools identify the highest values in a portfolio within a given radius—usually 200 to 400 metres. As computational power has increased over the past 10 to 15 years, so has the resolution at which these analyses may be undertaken.
The second use of terrorism modelling is scenario analysis, or simulating a single attack at a high-risk location. Models simulate the blast pressure of bombs, the dispersion of toxic chemicals, and the impact of 9/11-style aircraft attacks. They then calculate the damages and fatalities based on engineering estimates of building performance and epidemiological assessments of toxicity. Scenario analysis is useful in determining the potential damages that could arise from a large-scale act of terror. But it does not consider the probability of such attack.
The third use is for probabilistic terrorism modelling. This is the most robust type of analysis, which considers thousands of different attack scenarios and their relative likelihood.
Highly valuable but still controversial
insured properties is undisputed.
Critics continue to contend there is too little data on successful large-scale attacks to draw accurate conclusions; that human behaviour cannot be modelled successfully; and that the range of possible damages is so high that modelling terrorism risk provides minimal value.
These arguments had merit when terrorism modelling was a new science. But during the past decade the information available to modellers has increased to the point where the probabilistic components of today’s terrorism models have firm empirical underpinnings. Furthermore, the kinds of terrorist attacks that would result in significant insurable damages are not subject to the whims of human behaviour: they are carefully orchestrated acts requiring millions of dollars in funding, years of planning, and dozens of operatives.
Thus, they may be understood (and modelled) with a reasonable degree of confidence.
Some of the data used in assigning probability to terrorism events includes plot frequency, counterterrorism funding levels, terrorism court convictions, and past terrorist events, for which there is publicly available data on more than 100,000 historical attacks. Thanks to intelligence disclosures and high profile leaks over the past few years, detailed data on terrorism targeting preferences and counterintelligence capabilities has emerged. All of this information can be used to gain valuable insights into relative likelihood of various forms of terrorism. Very little of it was available 10 years ago.
Even if one chooses to dismiss the value of probability in terrorism modelling, there is still tremendous utility in the relative values that the models provide.
For example, if the model determines that one risk carries twice the loss potential as another, this relativity is driven by criteria such as proximity to high profile targets, susceptibility to different forms of attacks, and the overall risk level of the city. These criteria are rooted in empirical data, and the model results provide actionable value to underwriters and portfolio managers.
Pricing any kind of insurance risk—hurricane, earthquake, or terrorism—requires making an explicit assumption about the probability of a loss-causing event. Although terrorism models cannot draw from the same breadth of data available in climate science, there is enough information available to make reasonable assumptions about the damages and probabilities of large-scale acts of terror.
Insurers and reinsurers should make full use of this data. When combined with the advancements in computational power and modellers’ understanding of the engineering principles of terrorism events, the data can allow insurers to be more confident about writing higher volumes of business in risky urban territories.
The industry is in good shape
The global risk landscape is fluid and volatile, requiring models that are continually updated to reflect the global threat level.
The terrorism risk specialists at RMS continually monitor changes in the risk landscape, providing a granular view of terrorism risk to manage exposure, set corporate risk tolerances and assess the relative risk between prospective portfolios. By including a catalogue of more than 90,000 high-resolution attack scenarios and almost 10,000 terrorism targets it also provides deep insight into the drivers of loss underlying a portfolio. The high-resolution analyses provide a detailed view of the hazard and vulnerability across many different types of attacks.
Dr. Gordon Woo, a pioneer in terrorism modelling, has often said that in the US and Europe, terrorism insurance protects against the failure of counterintelligence, just as flood insurance protects against the failure of flood defences. With the increasing amount of data available on the extent of these counterintelligence capabilities, the insurance industry can underwrite and manage the risk with increasing effectiveness.