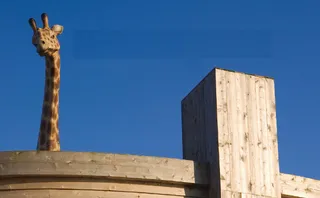
After the flood
According to Swiss Re’s preliminary estimates, flooding was the main driver of natural catastrophe-related losses globally in the first half of 2013. Floods caused an estimated $8 billion in insurance claims, making 2013 the second most expensive calendar year in terms of insured flood losses on Swiss Re’s sigma records, while AIR considers inland flooding responsible for $5 billion in losses a year in the US. But flooding—despite being a common occurrence all over the world—is notoriously difficult to model. Examining where current models fail is an important step in understanding the next generation of flood predictors—and getting a handle on how best to run them.
Iain Willis, flood products manager at EQECAT, explained, “Unlike other perils like windstorm or earthquakes—which often have more uniform patterns of destruction over large areas—floods are more polarised in their damage footprint.”
According to AIR Worldwide’s director of flood modelling, Dr Boyko Dodov, the US government creates another challenge in the quest to model North American flood risk. He said, “The first challenge to flood modellers is the market itself. Because of heavy involvement in the private market by the US government, the flood market has not been attractive for insurance pioneers and innovators.” This has stifled innovation and pushed the peril by the wayside.
To top it off is the constantly changing landscape of flood-prone areas: new developments and the increasing penetration of humans with their constantly evolving risk profile must be contended with. Brian Owens, senior director of model solutions for RMS, added, “There is greater insurance penetration in some of the markets in which we operate. A main driver in the change of loss is the amount of insured exposure in catastrophe-prone areas, which is increasing. A lot of this results directly from where we choose to live and locate our assets, and also how much insurance we buy to protect those assets.”
Ian Forwood, sales director at NIIT Insurance Technologies, has advice that sounds simpler than it is: “The industry needs to ask itself such as ‘What if this area floods?’ and ‘What if there’s a flood that is more severe than anything we have seen before?’”
The data problem
Models for many types of flooding exist. Everything from storm surge and rivers overflowing their banks to sprinklers breaking in an earthquake can be modelled. But as innovative as catastrophe models are, there’s one major challenge that the most creative thinking in the world hasn’t been able to get around: data. Or, rather, the lack thereof.“The quality of flood models is driven by the availability of good data,” Willis said. “For small areas with greater density of hydrological data we are able to construct models that accurately capture the flood risk in these systems. Conversely, in areas of sparse data or for very large physical areas, flood models sometimes struggle.”
Owens added, “In all cases flooding is a high-resolution peril. The level of flooding can vary greatly over short distances, and where it does occur, it can be extremely damaging. It differs from some of the other modelled perils in that sense. Accurately modelling flood requires high-resolution data and models that are able to accommodate that high-resolution data. A pretty complex set of components goes into a flood model.”
Modelling flood losses can be something of a moving target, particularly when the data don’t exist. Both Willis and Owens cited the central European floods as a significant event for the same reason: while similar events had occurred in the area before, this time around the flood defences were effective in preventing the flooding of major urban areas.
Willis said, “Although overall insured losses appear close to the 2002 flood event, it was noteworthy from a modelling perspective that damage patterns were often markedly different. Increased flood protection, residential take-up of insurance in high risk areas and many new defence schemes meant that damage patterns were somewhat surprising.”
Prevention techniques are a focus in the US as well, although AIR sees the need for a more nuanced approach. Dodov explained, “Understanding the condition and location of levees and other flood protection has been one of our focuses at AIR, in order to probabilistically model the risk behind flood protection structures. The binary approach to levees, for example, is that there is only risk beyond their design capacity and an event will be either below or above that level. Our model incorporates fragility curves for these defences based on all available data from the Federal Insurance and Mitigation Administration, US Army Corp of Engineers and the US Geological Survey because we understand that these defences can fail before reaching their design protection level or sustain peak flows above this level.”
Willis added, “As uncertainty becomes inherent, modelling assumptions have to be made. For example, in constructing our German and Austrian flood model, Euroflood, there was a substantial amount of historical data about the key river systems of this region. Trying to reduce the unknown variables is really the key action point for both modellers and reinsurers. If models are provided with detailed information on the location, occupancy, structural modifiers and construction types, we are in a much stronger position to accurately model a client’s risk.”
An end to uncertainty?
Removing uncertainty from modelling is easier said than done. Owens said, “We need to acknowledge that natural catastrophes are, by their very nature, uncertain, and consequently our understanding of risk will continue to evolve in the future. Flood is a good example of where learning is ongoing. The Thai floods in 2011 showed that, while we have made meaningful progress with flood models, flood risk remains an extremely complex physical system and is an inherently challenging peril to model. With the ongoing investments we make in the technology we use to run our models we continue to improve our representation of these complexities in our models.”
That being said, there are some steps that the modelling and reinsurance industries alike can take. Owens explained, “The quality and resolution of data being used in the market are improving. It’s important for modellers to be confident that the data they are using accurately represents their risk, and also that it’s as complete as possible.”
"Increased flood protection, residential take-up of insurance in high risk areas and many new defence schemes meant that damage patterns were somewhat surprising."
Forwood said, “Technology can be an enabler here, especially in the areas of geocoding, to make sure that flood exposure of an individual risk is assessed correctly, and proximity analysis, to determine the concentration and hence aggregation of exposures in respect of potential or actual events such as flood. Technology can also be used to provide cost-effective data on high value risks in emerging markets, which underwriters can use as part of a multi-model toolkit to gain a better understanding of exposures.”
According to RMS, it helps its clients to build in greater resiliency to their risk management strategies by enabling them to explore uncertainty through RMS models, offering greater options in terms of how individual clients understand, evaluate and use the models they buy. Owens said: “We’re supporting clients in their ability to be able to evaluate models and to validate that particular models are appropriate for their portfolios. That’s an important part of successfully modelling exposures—understanding and evaluating the models you’re using.”
Owens continued, “RMS(one), to be released in April 2014, is an open modelling environment, allowing reinsurers to run sensitivity tests, incorporate their own research and bring in models and model results from other providers. The open nature of RMS(one) will allow reinsurers to bring in models where an RMS option doesn’t exist or to get a second opinion on a particular risk.”
According to Willis, reinsurers should also rethink the way they go about collecting their data. While many use aggregate data, it may be the wrong way to make an accurate model. He said, “The problem of using such data is that before you even begin to model the exposure you have already introduced a tremendous amount of uncertainty. For example, if the postcode is quite large, and built-up or has significant changes in terrain, the variation in flood hazard may be significant within a single area. This uncertainty is greatly reduced if reinsurers have detailed coding data.”
EQECAT, he explained, can help. “We’ve introduced an alternative disaggregation option for reinsurers. A new function in RQE addresses the fact that insurance is not always written in the highest flood risk areas of Germany. If selected, the tool excludes the client’s exposure from the one-in-ten year flood zone and reallocates it outside of this area. In the absence of detailed data, we believe such techniques help clients manage their assets more successfully.”
Willis is confident about the future. “Modelling of flood hazards has significantly improved in recent years. The wider availability of hydrological data and high resolution digital terrain models enables us to produce flood footprints in increasingly more detail. However, although we’ve improved our understanding of the hazard, there continues to be a large amount of uncertainty around flood vulnerability.”
Challenges will remain. But collecting unparalleled data, validating the appropriateness of your model and looking at every loss as a lesson will go a long way towards pinning flood down in the future, and AIR believes the effort well worth it. Dodov concluded, “We anticipate that flood models will play the same critical role that hurricane and earthquake models currently play for insurers. There is a real market opportunity for insurers who use the new tools to innovate or capitalise on a potential shift of primary flood coverage to the private market.”