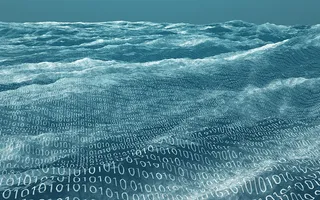
The importance of data in managing cat aggregation
The increasing severity of cat losses will make data granularity all the more pressing for reinsurers. Ben Miller, director, advisory at KPMG, talks us through the need for greater oversight of re/insurance exposures.
For Bermuda reinsurers—and indeed for the entire property casualty industry—2013 was a relatively benign year for catastrophe events. Property casualty industry net income and return on equity are estimated to both be at their highest levels since 2006. 2013 earnings results of the majority of Bermuda reinsurers were strong and exceeded analysts’ expectations.
Cat losses have become an increasingly significant component of loss for the property casualty industry. According to the Insurance Information Institute, the average cat loss component of the combined ratio has increased from roughly 1 point in the 1960s to 1980s, to roughly 3.5 points in the 1990s to 2000s to more than 6 points in the 2010s so far. Relying on low cat years in order to meet return on equity goals is becoming increasingly risky.
We have also entered an era of climate volatility and events occurring that modelling companies did not have in their event set, or which they had modelled, but were significantly underestimating the insured losses. 2011 and 2012 were the sixth and third highest years for inflation-adjusted cat losses in US history, with record numbers of tornadoes in 2011.
Examples of unusual and changing weather patterns abound: the US eastern seaboard’s ice and snowstorm barrage; the winter rainstorms and flooding in southern UK; and last July’s intense storms, “larger than tennis balls” hailstorm, and ensuing flooding in northern and southwestern Germany, to name a few. Despite the overall lower than average cat losses in 2013, there appears to be a pattern of increasing cats in recent years due to severe storms, and convective events such as tornadoes.
Is the Bermuda reinsurance industry prepared to weather the storm of bad cat years? And what are reinsurers doing about your portfolio to dig down into the detail and manage their catastrophe concentrations? It is helpful to keep in mind that as reinsurance market pricing and terms and conditions have softened, the quality of the underlying data has in some instances deteriorated. This is typical of softening rate environments. Reinsurers should benefit by digging into the detail—namely, managing and understanding the quality of their catastrophe exposure data and what that means for the modelled results, and knowing their underlying cedants’ exposures better.
The first suggestion is not to rely too heavily on model results. They are necessary, but not sufficient in managing cat concentrations. It is fine to review probabilistic and deterministic model outputs, but smart reinsurers look past the model results, and make sure they have a handle on exposure concentrations and the potential impact, if assumptions in the model are not correctly calibrated.
It is wise to focus on the largest and most exposed areas, the most significant events impacting the portfolio and what the potential losses could be. Consider the impact if the model you are trusting to make decisions proved to be off by 25 to 40 percent.
Of course, the company’s potential market share in a given event is important. Consider the possibility of different (modelled and unmodelled) events that would leave your company with a large loss of capital relative to peers, and contemplate whether you are getting paid enough for that risk. Think about how investors may react to you relative to your peers if that event were to happen.
Data quality
When it comes to your cedants’ data and their submissions, consider carefully the impact of changes in exposures in renewal contracts, particularly the cedants’ latest exposures, which may be less pristine in terms of data quality. For an insurer, new business tends to be the business where they have the least knowledge about potential exposures. Once the account has been on their books for several years, they are likely to have a much better handle on exposures, and have more accurate and complete exposure data, but should be wary of the data quality and completeness of large new accounts in their cedants’ exposure data sets.
Enquire about and attempt to measure the extent of missing or underreported data by cedants. Underreported exposures can include locations that are not being geo-coded at a high geo-coding resolution or at all, or are too small for the cedant to obtain them from the underlying insured. Having many small, unknown exposures in a major city that is already highly represented in your known exposures can lead to unwelcome surprises if an event occurs.
If they are prudently managing their own cat concentrations, cedants should be able to provide information on missing (residual) exposures and what percentage of their portfolio these represent in, for example, major urban areas. The potential impact of a number of smaller to medium-sized unreported exposures in this area could be significant in deciding whether to retrocede exposure.
Geo-coding resolution and accuracy, and construction data availability and quality are very important. A location that is geo-coded to the centre of a zip code can, in many cases, be geo-coded quite far from its actual location. Vendor cat models can be quite sensitive to construction type and height, and in deterministic and probabilistic modelling results may treat unreported, or unknown, construction information more favourably than if the actual construction information were available to include in the model’s exposure data set.
It is not unreasonable to make requests of your cedants for reports that focus on the reliability of their portfolio construction information by industry or area. Many insurers are doing this to quickly identify issues like steel frame high rise structures reported for big box retailers or in rural areas. Modelling error can be significant.
It is good to enquire about how aggressively insurers manage their concentrations internally. You should be able to get a strong sense of their commitment to being serious about their cat exposure management. Be wary of the extent to which insurers override the modellers’ geo-coding engine and plot locations themselves. This may be done using tools such as Google Earth to move the locations to a more correct spot on the map and thus, improve the accuracy of the data. On the other hand, it may be done by tweaking location data internally to stay below management capacity constraints, particularly in high concentration areas.
When it comes to non-property reinsurance such as workers’ compensation, be aware of portfolios that have a high level of mobile exposures. Engineering contractors that frequently work on the premises of chemical refineries is one example. A mobile workforce can be significantly in harm’s way and be unknowingly adding significant exposures to existing, known property exposures. Workers travelling temporarily to dangerous areas around the world may also be a source of significant unknown exposure.
Top models
Once you have done these data quality checks at the cedant level and you have attempted to get the clearest understanding of your cedants’ exposure, consider ways to adjust cedants’ modelled, expected loss and risk contribution to your portfolio based on the quality of their data. One cedant may model better than another, in part because of differences in data quality. The models pick up certain elements of data quality in their standard deviation and other metrics, but not all. Market dynamics and relationships are critical, but the more informed you are, the better decisions you can make and the better prepared you are to optimise capital for maximum expected returns.
As far as the modelling results go—and what is and is not modelled—consider the potential impact of multiple events impacting the same or proximate exposures. For example, a swarm attack such as the Mumbai hotels terrorist attacks is a good example of what is extremely difficult to model and can impact a broader footprint than cat modellers currently reflect when modelling non-nuclear-biological-chemical-radiological terrorist events.
The cumulative impact of the extended rainstorms in the UK and multiple snow and ice storms that have hit the eastern US this winter resulted in losses greater than was modelled. In cases like these, losses to life and property can be increased due to insufficient government funding for, and availability of, clean-up and rescue resources and the cumulative impact on infrastructure including power, food and water supply chains.
High quality geo-coding and construction data are important and minimise the amount of uncertainty in your modelling results. Anticipating that the trend towards more frequent and severe catastrophe events rather than less is likely, the need for quality data and a granular understanding of catastrophe exposure is essential.