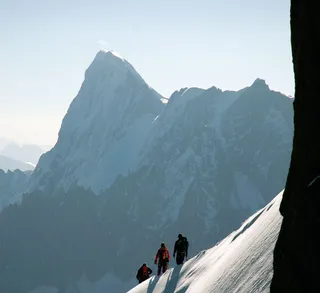
Modellers: an essential addition to your ILS team
Insurance-linked securities (ILS) are built on a sound economic principle: thanks to diversification, uncorrelated insurance risks all but disappear if divided up and taken by large pension funds who limit their exposure to a few percent of assets. But key to this is that fund managers should not have to understand the nuances of how insurers underwrite and pay claims. They would rather take a bet on the whole industry losing money (industry loss triggers) or a specific bet on the wind blowing or the ground shaking (parametric triggers).
So, the ILS market developed around trigger types that allow fund managers to take on systemic catastrophe risk without exposure to the idiosyncratic risk of individual companies. This creates new challenges:
• How can you design structures that mimic the reinsurance they replace?
• How can you design a trigger that can be modelled accurately?
• How can you ensure that you have all possible outcomes covered in language that describes how to settle the bond?
While the broker or the banker has expertise in understanding transaction motivation, the modeller has detailed knowledge of both the models used to measure the risk and the devices and techniques used to measure wind speed and ground motion.
Triggers should work in stressed ‘postevent’ conditions
Modelling firms have deep experience in what happens following an event, and in knowing how hazard measurement networks work. As such, they are able to design triggers that will work as intended in stressed ‘post-event’ conditions.Many deals use a reporting agency that provides data to determine event occurrence following a catastrophe. These agencies are often not contracted, but exist for the purposes of public data provision and disaster risk management more generally—so have no obligation to the transaction.
Reporting networks also have critical lifeline dependencies for their functioning. As seen in the Great Tohuku Earthquake of 2011, the K-net network of earthquake measuring stations failed to report data for a large number of stations following the earthquake, due to power and communication failure during the tsunami.
One transaction, Muteki, was exposed to this, due to the procedures in place to deal with failing stations. If a seismometer failed to report, it was to be treated as zero—a conservative approach favourable to investors, but not in the best interests of the sponsor (Zenkyoren). It took several weeks for the data to become available, during which time the deal was either untouched if no data were found, or a full loss if all anticipated recordings surfaced. Any investor buying Muteki in the secondary market at this point was essentially gambling that the K-net network had failed.
In contrast, other transactions exposed to the earthquake included steps to approximate missing readings through interpolation, rather than assuming zero readings. While it is conservative to assume zero values upon failure, it is arguably in conflict with the transaction motives, since larger earthquake ground motions are more likely to cause failure.
In these cases using interpolated values, the likely index outcomes were much clearer, and secondary market prices reflected this.
Design triggers to minimise subjectivity
For both issuer and investor, the last thing you want is for the payout on the bond to come down to a subjective decision from the calculation agent. The design of a sound trigger should remove subjectivity to every extent possible.
There may be explicit trigger components that require a subjective assessment, such as the interpretation of a description for translation into an index determination. This is perhaps best illustrated with an example.
The recent Mariah Re transaction used a concept of ‘metro factors’. Each covered state had two factors—one as standard, and one metro factor to be used if certain counties were named within a PCS bulletin. This was intended to allow different payout factors if certain metropolitan counties were impacted by tornadoes. The idea was that PCS would describe damage in its reports, and if certain counties were mentioned, this implied significant damage, and that the metro factor was appropriate.
However, an examination of past descriptions revealed subjective statements such as “the road between X and Y was blocked by treefall ...”—does this imply significant damage in either county?
In the end, Mariah Re was deemed a total loss when a PCS bulletin was updated to include a list of hailstone sizes by county. This led to the selection of metro factors that increased the index value beyond exhaustion. The bond paid out the full $100 million, in spite of lobbying from investors.
Another common interpretation is for event parameters. Many transactions rely on the calculation agent to calculate a modelled loss that might trigger the deal. Commonly, this uses public data, from government bodies such as the National Hurricane Center (NHC) or the US Geological Survey (USGS) (see table below).
However, these bodies do not report parameters explicitly for such purposes, so may not always produce them, or may publish ‘experimental’ values.
Issuers and investors alike must be comfortable with any subjective steps required, and the best efforts of the calculation agent if these are unavailable.
Design parametric indices that behave as expected
Cat bond triggers needn’t be complex. However, it is common to see relative over-specification, as sponsors attempt to minimise basis risk. The key here is understanding index response. A parametric index is designed to correlate to the sponsor’s loss experience, which is approximated using a model.The index is therefore akin to the vulnerability model.
A common index form is therefore a power-function of hazard values, representing a convex relationship between hazard and loss (see Figure 1).
This shape means higher rates of change at higher index values, which leads to significant volatility if it is not controlled. For example, there have been recent transactions where an increase of 1-2m/s at specific wind speed recording stations beyond a certain point would be enough to cause attachment and exhaustion. Arguably, the measurement upon which this depends is sensitive enough that such a difference could be down to recording error.
As these structural issues became more apparent, some transactions took index functions to the opposite extreme, applying limits to ensure that a bond cannot be triggered by readings at a single station. If done well, this can limit the index volatility while allowing the sponsor a hedge with limited basis risk. However, if done too aggressively, this can lead to an index that looks like a very attractive investment—a muted index may respond so slowly as to appear highly unlikely that it will ever trigger, requiring implausible event scenarios to do so. Onesuch example occurred where a vast number of wind speed recording stations had to measure extreme wind speeds in order that the deal could trigger. In any model other than the one to which this was tuned, such a scenario was all but impossible.
This concept leads us to another important consideration under the topic of index volatility, which is the degree to which the index is fitted to the model. Over-reliance on the model is likely to lead to an index that will behave unexpectedly if scenarios not contemplated by the model unfold.
An index with many terms, the values of which are driven by a handful of dominant terms, is a sure-fire indication that the index is over-fitted, as are index functions that appear to have come straight out of a regression analysis of modelled event values.
Understand how resets can change the risk of the bond
Resets perform two functions. First, they should keep the risk in the transaction constant, to ensure that the risk doesn’t grow as underlying portfolios shift or as science evolves and models change. Second, they should allow sponsors to update the transaction, so that it continues to match the underlying exposures.
Many transactions include limitations on how much the risk can change during a reset. However, these are not always entirely robust, and can be manipulated. For instance, where exchange rates are included in a transaction, but are not stipulated by an external source, these can be used to manipulate concentrations. Setting an unrealistic exchange rate might allow a sponsor to shift the risk from one region to another.
Similarly, resets might give constraints across large regions (Europe being an example), but allow heavy concentration within that region. One such example occurred in Eurus II, where Hanover Re was able to update a pan-European cat bond, to become a northern Germany deal following an AIR model change.
Such concentrations can dramatically increase transaction volatility.
Help issuers and investors build intuition
Investors and sponsors both benefit from analyses and scenarios to develop intuition on when a catastrophe bond would trigger. A good modeller will provide insightful analyses that bring a cat bond to life.
Historical analyses
Cat bonds are relatively unique as an asset class, as they include expert risk analysis performed by a third party modelling firm. This will contain an examination of historical events, to help give context around potential past performance. It is often useful to make a quick check of the number of triggering scenarios within a given time period, and ensure that thisfits with expectation. Whilst this is a very rough way to understand the risk in the transaction, it can be useful to gain quick insights and understand where something unexpected might be happening.
Industry comparisons
Similarly, a comparison against industry loss values for these historical events, and for other modelled scenarios that might be disclosed, can help gauge bond behaviour. For example, a comparison of industry loss values against transaction index values will help underline where a bond may exhibit unique properties. This may also be a useful indication that the bond is not strongly correlated to other cat bond investments you might hold.
Index insights
Many indices can be inverted, to give the attachment point as a function of hazard values. This allows simple and intuitive calculations as to the levels of hazard required to cause loss.
For example, an index function: I = wi (vi - v0)3
Where wi is the station weight, vi is the station hazard, and v0 is some minimum hazard reading required, can be inverted to give:
v = v0 + (I / w)1/3
Where I is now the required index value (perhaps attachment), w is the amount of the weight impacted by the hazard value, v.
Scenarios
You can also use an index function to test scenarios that might not be included in the risk analysis. It can be of benefit to develop a range of scenarios against which you can test all bonds in your portfolio. Perhaps you are concerned with a $100 billion Florida hurricane—any PCS-index can quickly be tested against this scenario by applying the Florida share factors to infer an index value.
Similarly, it can be useful to apply unrealistic scenarios to gauge bond performance, for example asking the question ‘what if every station between 40 and 45 degrees latitude were impacted by 30m/s wind speeds?’ This will soon expose concentrations of risk that can otherwise be difficult to spot, and is easily constructed in a quick spreadsheet analysis.
The recent strong growth in the ILS market suggests that more and more issuers and investors are discovering the merits of this asset class. But an investor holding a market-weighted portfolio of ILS in 2011 would have returned less than 2 percent above LIBOR. An investor who had spotted the structural deficiencies of Muteki and Mariah and avoided them would have returned well over 10 percent.
We believe that healthy growth in this market will require all parties to understand that trigger design and deal structure are both vitally important to ensure a bond behaves as expected..