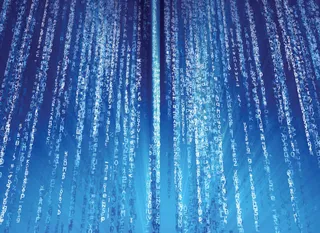
Data management: take advantage of the regulatory burden
As reinsurers continue to refi ne their approach to risk-based capital, chief information offi cers (CIOs) and other executives are being faced with increasing challenges in the realm of data quality management. For some time CIOs have been increasingly pressured by boards, rating agencies, investors, capital markets and clients for more information, delivered at a greater frequency and that is more accurate and granular. CIOs can now add the regulator to this list of stakeholders who require more information.
Indeed, systems architecture and data management should be a strategic agenda for those who want to differentiate themselves from the pack, understand the impact of volatile markets and react ahead of the competition. Regulatory drivers – specifically Solvency II and equivalence – are creating a burning platform for data quality, but companies should be building these requirements into an overall strategic plan rather than merely reacting to them with a compliance mindset.
The new regulatory regime introduces de facto requirements on data quality and reporting, and some reinsurance companies will be challenged to comply. These data standards are not limited to the quantitative requirements of Pillar 1, where good quality data is key to producing quality capital calculations, but also apply to Pillar 2 and Pillar 3.
Pillar 2 requirements are concerned with governance and supervision and while seen as more qualitative than Pillar 1, companies are beginning to realise that proving good governance and husbandry is diffi cult to provide evidence for, unless reliable quantitative measurements are available. These measurements, primarily of risk, must then be used in conjunction with the data gathered in Pillar 1 as part of the company’s Commercial Insurer’s Solvency Self Assessment (CISSA) process.
A core element of the Pillar 2 requirements is being able to demonstrate that there are appropriate management controls over the data used to calculate capital requirements. To date, the focus on data quality has been driven by Pillar 1 elements such as fi nancial reporting and data and Pillar 2 has been overlooked.
Guidance on the data robustness requirements is still uncertain and many organisations in the interim are struggling to identify where the gaps exist. Data quality assessments will need to be completed soon, or time will run out to complete the necessary data remediation and process implementations. The dynamic nature of the industry combined with the different operating models of the various organisations will always mean that it is difficult to determine whether there is a common standard that will cover the entire spectrum.
Companies have yet to receive a great deal of clarity around the BMA’s thinking in respect of the Pillar 3 reporting requirements. Nevertheless, companies should start assessing now what the challenges are likely to be. Business units and fi nance should be driving the dataagenda, and working with IT departments, as this is not an IT-only issue. Questions which need to be anticipated include:
• Is the company currently producing data at a sufficiently detailed level?
• Are there consistent data standards across the group?
• Can disparate systems produce data in a consistent format?
• Who are my reporting audience (board, investors, rating agencies, regulators, management) and are there common requirements?
• Can we deliver on increased reporting frequency, shorter turnaround and ad hoc reporting?
• Are there still too many spreadsheets and manual processes?
• Can my outsource providers (custodians/asset managers/TPAs) deliver on these evolving requirements, and are they reliable?
• Are reporting systems fit for purpose?
Many companies will be satisfied with their responses to some of these questions, but it is unlikely that most will be satisfied with their response to all of them. Some will find that there is an abundance of data available but it is not in the correct format, is siloed or cannot be produced in a timely manner; others will discover that data is insufficient to meet current or future needs and cannot be reported upon consistently.
Providing quality data: the regulator’s view
The risk-based capital regime requires internal policies and procedures for the management of data throughout its life cycle (identification, collection, storage, processing and archiving). Ideally data should be owned by the business and not by IT, and data management should therefore be implemented across all applicable areas of the organisation. Before discussing the importance of data, there is a need to define ‘data’ within the correct context. In the absence of a clear steer from the BMA, the Committee of European Insurance and Occupational Pension Supervisors (CEIOPS), the predecessor of the new European Regulator (EIOPA), has classified data as “information required to complete a valuation of technical provisions, specifically using appropriate actuarial analyses and statistical methodologies”.
CEIOPS also recognised that there is no point in categorising data if there are no guidelines that enforce quality control. As part of an organisation’s move toward basic solvency capital requirements (BSCR) data compliance, there is a requirement for various impact analyses and data quality assessments. Without conducting such an assessment companies are prone to ‘garbage in, garbage out’.
As a universal rule of thumb, the quality of data should be determined based on its:
• Appropriateness
• Completeness
• Accuracy
Appropriateness
Data must be relevant to the risk being carried and the capital calculation required to cover the total exposure. This may require the firm to adapt its data standards to the types of business that it underwrites. Data that is generally accepted as appropriate will be 1) suitable for the valuation of technical provisions, 2) relate directly to the underlying risk portfolio, and 3) be sufficiently granular.
Completeness
Under the BSCR, data is generally considered complete when 1) the data covers the main homogeneous risk groups in the liabilities’ portfolio, 2) the data has sufficient granularity to enable full understanding of the behaviour of the underlying risk and the identification of trends, and 3) the data provides sufficient historical information. There is usually a thin line between completeness and oversaturation, but the best starting point is to have more than you need and rationalise as appropriate.
Accuracy
Data is accurate when 1) integrity is not diminished through errors or omissions, 2) data is stored and retained adequately, 3) a high level of confidence can be placed in it, and 4) data can be demonstrated as credible by being used through the operations and decision-making process.
Reinsurers will be required to establish their own concept of data quality as part of creating their data policy, and develop rules to determine how these key attributes are judged. They must also develop their own tests for data quality and apply them to monitor and report on the quality of data. Quality reporting forms part of the standard regulatory submission package, so methods for effectively gathering and presenting information on data quality must be developed. The frequency with which these assessments are to be made has led many organisations to consider automated testing approaches based on a centralised library of business rules with which the data should comply. These rules may then be built into process control frameworks to gain further efficiency and get data right the first time.
Many readers will be familiar with the statement on auditing standards (SAS) 70 auditing standard, which requires service organisations and service providers to demonstrate that they have adequate controls and safeguards over hosted data or data processing. Companies should consider whether a similar approach should be taken to their own data and consider how they would fare if held to the same standard.
Identifying data deficiencies
When the data analysis is done and the dust has settled, what’s next? Identifying data deficiencies can be considered one of the most important exercises in an organisation’s BSCR data cleanup efforts. Deficiencies in the areas of appropriateness, completeness and accuracy form the basis of why such stringent regulations on the quality of data were enacted in the first place. When the assessment of data quality returns negative results, an organisation must take the following into consideration:
• The nature and size of the portfolio
• Deficiencies derived from the handling of data (collection, storage and quality valuation)
• The lack of a reliable, standardised way of exchanging information.
Simply put, collection methods and calculations must be scaled appropriately for them to be considered effective. When there is insufficient data accurately to produce statistics and benchmarks, it becomes impossible to rely on such methods. This can also be caused by anomalies in data collection (for example, issues such as business interruption and seasonal trends). Second, if the collection method is fatally flawed, the data, and ultimately the reporting, will suffer greatly.
If such a deficiency in data collection, storage, or quality valuation is evident, the rectification responsibilities will usually fall in the hands of the CIO and the IT department. This means time spent with programmers, developers, and application specialists to get the most out of the data and reporting. Alternatively, investments will need to be made in tailoring an effective data collection process. Finally, templates should be created using best practices to determine the most effective way to exchange information with business partners. These templates should be highly standardised and robust enough to cover all bases.
Establishing data quality standards: new solutions
In many cases, efforts to define and assess quality data have lagged behind other work streams due the inherent dependencies on architecture, methodology and process. As work streams havedeveloped and platforms have been established, data work has started to move forward. The main areas of activity have been around finalising and implementing data governance frameworks, continued building of data dictionaries, data quality rule libraries, and data quality tool selection. A well defined data dictionary/ directory forms the basis of all future data quality assessments. Data definition can be accelerated by using data profiling tools, but it is necessary manually to identify business owners for all data elements and assign responsibilities for the quality, update, and timely provision of the data.
Consideration must also be given to the format of the data directory to ensure that it can be maintained and used efficiently by the business. An ideal solution for the directory is to build it into the core data flow, so that changes or errors are automatically identified and reported. The BMA makes no specific requirements of such systems, but existing systems may be unable to support the faster reporting and increased data governance/control required for compliance. Many companies are choosing to implement new data warehouse solutions to gain greater control over data sourcing and storage, and to demonstrate to the BMA that their data can be relied upon.
Data done right
So how do we get ‘data done right’? Ensuring success involves assessing the stability of existing data practices against the business needs, while implementing remediation solutions and focusing on best practices going forward. Comprehensive tests and evaluations of data quality should provide ongoing assurance over the appropriateness, completeness, and accuracy of external data.
Although we have focused primarily on data requirements driven by regulation, companies should not lose focus on the main reason why data is important: managing the business and being able to articulate to stakeholders how and why you are different from, or better than, the competition. A company that puts business needs at the heart of their work on data will be the firms that will best be able to leverage their efforts for competitive advantage. CIOs should view changes in regulation as providing the opportunity to drive change forward. Companies whose sole focus is satisfying a regulatory requirement or checking a box will ultimately not see an appropriate reward for what is a considerable effort both in monetary terms and in the amount of management time expended.
Data plays a vital role both in business and regulatory terms and an integrated approach must be taken to ensure the industry is ready to deal with the increasingly onerous demands being placed on it by all its stakeholders. Extensive use of spreadsheets, manual processes and messaging with slick Powerpoint presentations is not sustainable.
David Ciera is senior manager, Solvency II advisory at KPMG Advisory. He can be contacted at: davidciera@kpmg.bm